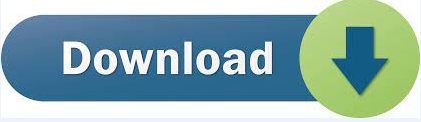
Check the render with menu bar > arnold > open arnold render view > button play / stop - Go to Hypershade * tab > aiStandardSurface * Outliner : select file1_1 * aiStandardSurface : RMB > assign to selected objet * aiStandardSurface : attribute editor : base > weight = 1, specular > weight = 0, geometry > opacity = 1 * TAB > particleSamplerInfo. Attribute editor: * Part "Sydomelight attributes": ¤ color = white ¤ intensity = 5 * Part Visibility: ¤ Camera = 0 - Create a cam and position it - Select objet file1_1 and in the attribute editor > file1_1Shape > * Part "Arnold" > radius multiplier > 0.1 * Part "Particle size" > only if needed : radius = 0.05. Not easy!! Here's how I did it : In Maya: - Have Hou Engine installed - Import asset hda - Play, check if it works - Create a light with menubar > arnold > light > sky dome. Our approach obtains high-quality tetrahedral meshes computed directly from noisy point clouds, and is the first to showcase high-quality 3D results using only a single image as input.So I had to dig more to find the solution.
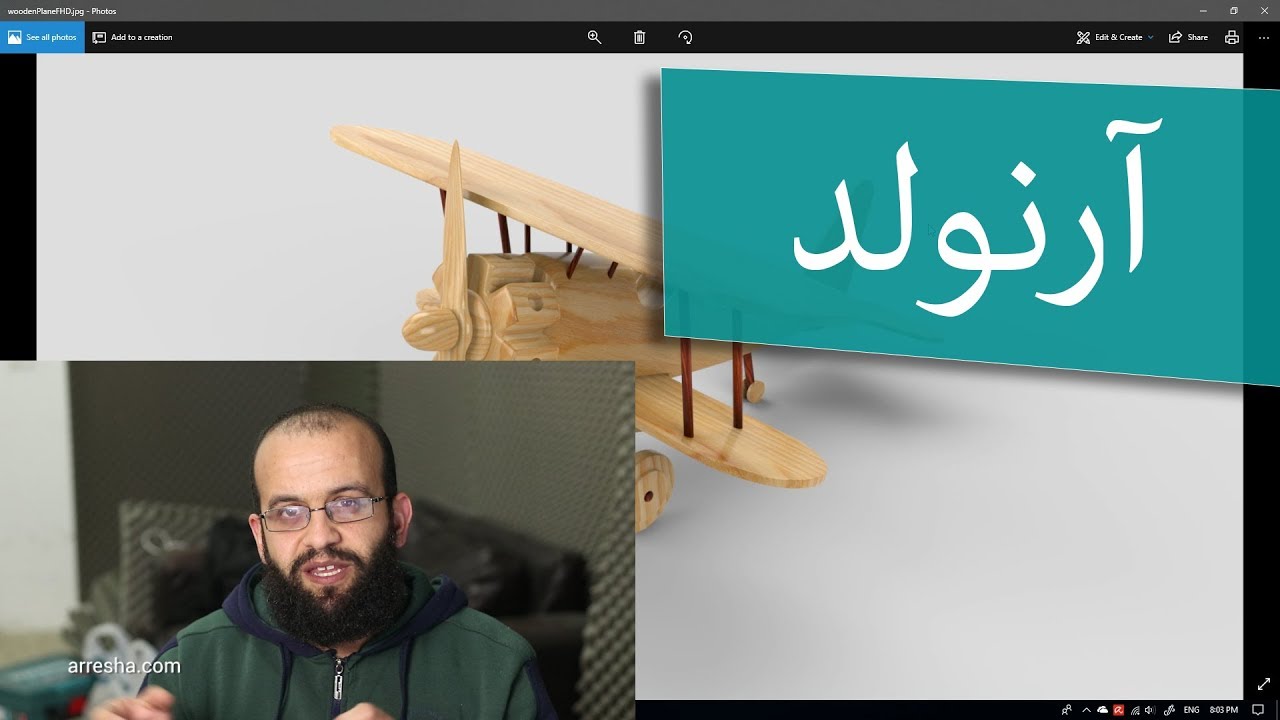
We demonstrate that DefTetmatches or exceeds both the quality of the previous best approaches and the performance of the fastest ones. The predicted surfaces are also inherently defined as tetrahedral meshes, thus do not require post-processing. We show that it can represent arbitrary, complex topology, is both memory and computationally efficient, and can produce high-fidelity reconstructions with a significantly smaller grid size than alternative volumetric approaches. It is thus simultaneously high-precision, volumetric, and amenable to learning-based neural architectures.
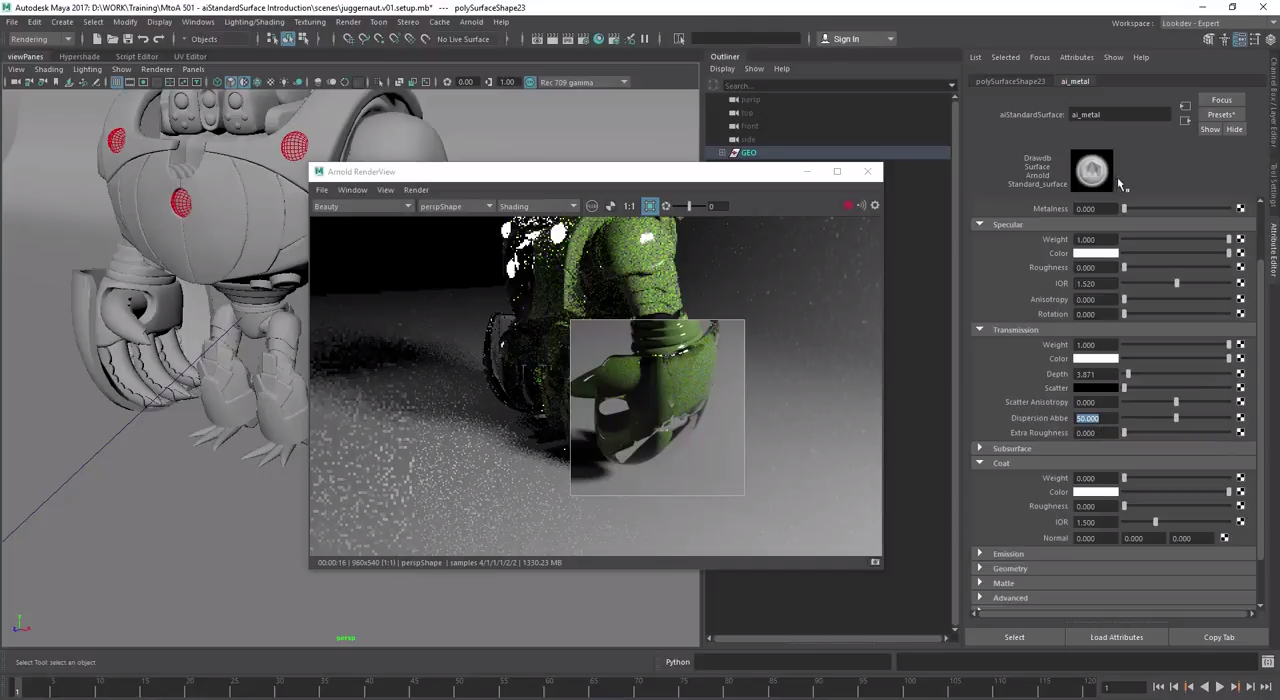
Unlike existing volumetric approaches, DefTet optimizes for both vertex placement and occupancy, and is differentiable with respect to standard 3D reconstruction loss functions. We introduce mph (DefTet) as a particular parameterization that utilizes volumetric tetrahedral meshes for the reconstruction problem. Previous work on neural 3D reconstruction demonstrated benefits, but also limitations, of point cloud, voxel, surface mesh, and implicit function representations. 3D shape representations that accommodate learning-based 3D reconstruction are an open problem in machine learning and computer graphics.
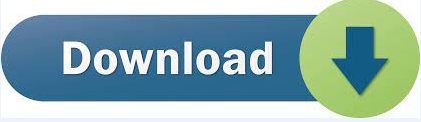